Artificial Intelligence in Senior Care
Any glance at the transcripts of quarterly earnings calls of publicly traded companies over the last few years shows that AI went from something casually mentioned by tech companies to something discussed increasingly in depth by hundreds of companies across numerous industries.¹ Salesforce even started having an AI engine “attend” its executive meetings last year, where it reportedly called out people for questionable forecasts and the like.²
Put simply, everyone knows AI is going to be really important, but most executives don’t seem to understand how. Quant guys (quantitative analysts) only have to mention any type of applied mathematics or quantitative analyses they want to do is in fact AI and – like magic! – they get funded – just so the executives can say they’re “doing AI.”
Before we get into how artificial intelligence impacts senior care, it’s best to start with an understanding of what it is in practice. The short version is there are three main types of AI: Artificial Super Intelligence (ASI), Artificial General Intelligence (AGI) and Artificial Narrow Intelligence (ANI).
ASI is the holy grail to researchers in this field and by all indications is a long way off. Basically, ASI means a form of non-human intelligence that can outperform humans in pretty much anything that we currently do, and in some cases by a very wide margin. This is the sci-fi stuff you see in movies that either results in utopian or dystopian futures. Think Westworld, Ex Machina, Her, etc. AGI means a non-human intelligence that is equivalent to anything humans can do, but isn’t better at those things than we are. That’s also not around the corner. Finally, ANI is where the world is mostly pushing into now. It’s basically a type of intelligence that can do one type of thing that humans can do as well as or better than them, but is limited to that specific application. That’s the type of AI we’ll see making an impact in senior care in the short- to medium-term.
Examples of ANI are all around us. Most of the time we don’t really give them much thought. But all of those examples share a general similarity. That is, a form of artificial intelligence that ingests data from the world around it (via a spreadsheet, a sensor, a camera, etc.), analyzes it based upon algorithms (basically increasingly complex “if-then” logic) that it has been taught (or has taught itself), and then makes a decision and acts independently of a human. In virtually all these cases, the ANI is continuously learning and getting better, meaning that it never forgets and never makes the same mistake twice.
Online shopping is a great example. In the past, someone would analyze what people shop for and what they are likely to buy in conjunction with that item or service. Then they’d set up some sort of campaign or action to drive that person toward making that additional purchase. ANI is much better at this than we are. Every time you see a suggestion for a product and it seems like they read your mind, that’s ANI at work. And every time you click or don’t click it is learning and getting even better.
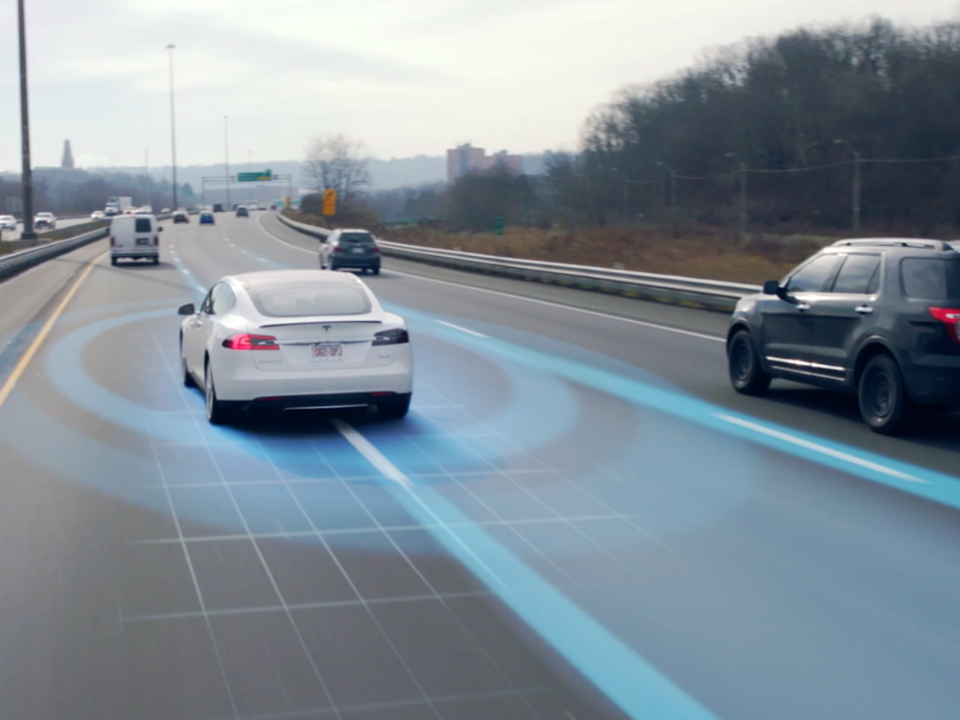
Now that we have a pretty good understanding of AI – more specifically ANI – let’s talk about the role it can play in two very large and pressing issues in Senior Living: labor and outcomes.
Using Artificial Intelligence to Get More Done with a Leaner Staff
I’ve spoken to hundreds of Senior Living providers this year, and every single one has identified personnel shortages as a major issue, with some even closing communities because they cannot keep them adequately staffed. Add to that the ongoing shift toward higher acuity patients and residents in these communities and something has to give.
Now, we’re a long way off from robots staffing buildings in any general capacity and flat-out replacing people. Japan is only experimenting with this in a very limited capacity for very specific tasks. However, ANI can start to transform the way that care is delivered – even who delivers it.
Take resident monitoring, for example. We all know that staff in these communities already feel overstressed and overworked. They absolutely cannot be everywhere at once, checking in continuously on residents (some of whom don’t want to be disturbed in the first place) and so on. But they don’t need to be. There are scores of solutions being developed and tested as we speak that envision a better way to passively monitor residents utilizing technologies like wearable sensors, UWB radar and machine vision to train AI engines.
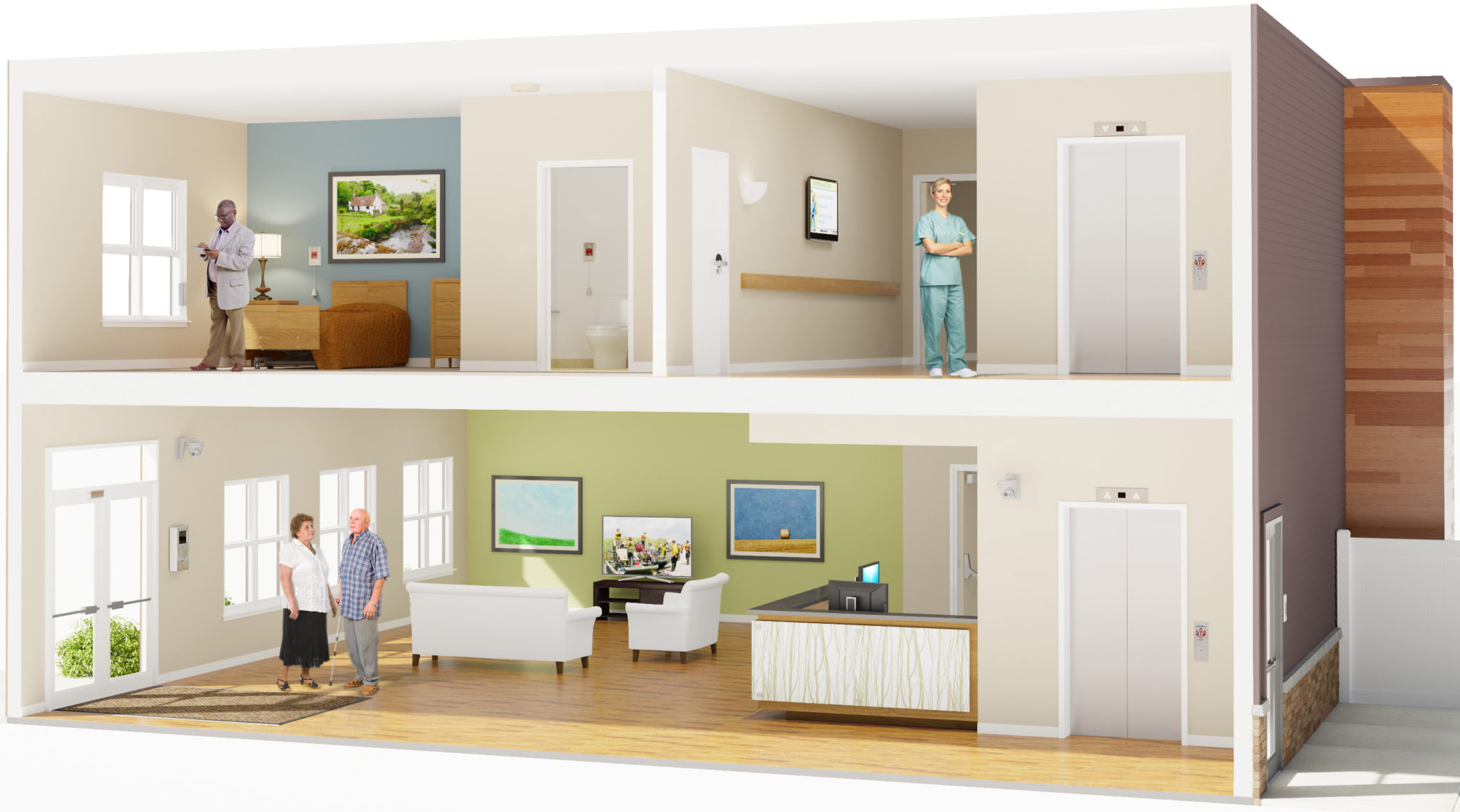
Contrast that scenario with the current standard of having caregivers that are in short supply checking in with residents around the building, potentially doing balance assessments at some interval and hoping to discover a change in gait/balance before it translates into a fall. And then a PT (which, by the way, just became the sixth-hardest position to hire for in the US) attempting to do one-on-one rehab with increasing numbers of people, and you can see how this can rapidly remake how we view care delivery.
Then, there are the legions of caregivers executing mundane tasks like scheduling and billing. All of those tasks could largely be addressed through the sensible deployment of Robotic Process Automation (a basic form of ANI), thereby freeing up those caregivers to fill more meaningful and fulfilling gaps elsewhere in a community.
Then, there are the legions of caregivers executing mundane tasks like scheduling and billing. All of those tasks could largely be addressed through the sensible deployment of Robotic Process Automation (a basic form of ANI), thereby freeing up those caregivers to fill more meaningful and fulfilling gaps elsewhere in a community.
Delivering Quantifiably Improved Outcomes Through Artificial Intelligence
The transition to value-based care from standard fee-for-service has been slow but inevitable. In 2016, the US crossed the threshold of 18% of GDP being attributed to healthcare (compared to under 5% in the early 70s) and that number is still growing.³ And here again is where ANI can play a pivotal role. The deployment of EMR systems harkens back all the way to the early 1970s, but for a host of reasons beyond the scope of this blog, its full potential has been far from realized. Still, the ability to coordinate care delivery within an acute or outpatient care setting is light years beyond where it was even ten years ago. Senior care providers continue to follow their acute care colleagues with the implementation of this technology. As more communities coordinate with hospitals and other players, opportunities for ANI to assist in optimizing care delivery and outcomes are legion.
Imagine a SNF with 100 beds. The conditions of the patients being treated there span a wide range. But in the eyes of the administrator and the clinicians in that facility, there is a clear goal: treat those patients in such a fashion as to prevent them from rehospitalization. Even with 100 clinicians constantly running from room to room checking vitals, pouring over notes and reading discharge files, this is next to impossible given the level of acuity now addressed in SNFs. ANI, on the other hand, can multiply their efforts.
At this very moment, scientists, engineers, and clinicians hard at work developing ANI-driven solutions that can do the work of an army of caregivers. Picture an RN about to do rounds. She and her team know of a few high-risk patients in the facility, and they will visit them first. Beyond those patients, however, everything else is just an educated guess with the information at hand (go to room 301, then 302, 303, etc.). But what if those patients were being monitored by an ANI engine?
I spoke with an entrepreneur who has already built an ANI engine that has absorbed hundreds upon hundreds of thousands of patient days and tens upon tens of thousands of clinical diagnoses. The result: instead of guesswork during rounds, that same nurse would know to go and check in on Mrs. Jones in room 213. According to the data, she shows early signs of CHF, and the clinicians need to intercede now in order to prevent a rehospitalization. How does the ANI engine know this? Because it reads and understands the text fields from the notes taken at 2:30 am and knows that Mrs. Jones complained of abdominal bloating. Delving into the vitals fields in the EMR for the past three days, it observes that Mrs. Jones has gained 5.4 pounds in the last 28 hours despite the fact that she only weighs 112 pounds. In other words, through the magic of Bayesian mathematics and Markov chains, she has congestive heart failure. The RN just doesn’t know it yet, but by acting now she can prevent a rehospitalization.
- https://venturebeat.com/2017/09/07/buzzword-or-not-ai-is-now-a-thing-in-corporate-earnings-calls
- https://www.ciodive.com/news/ai-sits-in-on-salesforces-board-meetings-and-has-something-to-say/515606
- https://www.healthsystemtracker.org/chart-collection/health-spending-u-s-compare-countries/#item-u-s-health-spending-growth-higher-1980s-similar-since